Explainable AI vs. Black Box Models: Understanding Transparency and Trust in AI
The battle between Explainable AI (XAI) and black box models represents a critical turning point in artificial intelligence. While black box models operate as mysterious systems that provide decisions without explanation, XAI offers a refreshing alternative by showing us exactly how and why AI makes specific choices. This fundamental difference shapes how we interact with and trust AI systems in our daily lives.
Imagine having a doctor who makes decisions about your health but can’t explain why. That’s essentially how black box AI models work – they provide outputs without revealing their reasoning. In contrast, XAI acts more like a transparent medical professional who carefully explains each diagnosis and treatment recommendation, building trust through clear communication and understanding.
The stakes couldn’t be higher. In fields like healthcare, finance, and criminal justice, understanding why AI makes certain decisions isn’t just helpful – it’s crucial. A recent study from MIT revealed that in many high-stakes applications, interpretable AI models can achieve the same level of accuracy as their black box counterparts, challenging the common belief that we must sacrifice transparency for performance.
This article explores how XAI transforms the landscape of artificial intelligence by providing transparency in decision-making processes. We’ll examine real-world applications where interpretable AI makes a difference, uncover the limitations of black box systems, and understand why transparency in AI isn’t just an option – it’s becoming a necessity in our increasingly AI-driven world.
Main Takeaways:
- XAI provides clear explanations for AI decisions while maintaining high accuracy
- Black box models can mask critical flaws in their decision-making process
- Transparency builds trust and enables better collaboration between humans and AI
- The future of AI depends on balancing powerful capabilities with clear accountability
Importance of Explainable AI
Understanding how artificial intelligence makes decisions is essential in high-stakes fields like healthcare and finance. Explainable AI (XAI) acts as a window into these complex AI systems, showing professionals exactly how their AI tools reach specific conclusions.
In healthcare, doctors need to understand why an AI system flags a medical scan as potentially showing cancer. As noted in a comprehensive medical study, XAI helps physicians trust AI-driven diagnoses by revealing the specific features in medical images that led to the system’s conclusion. This transparency allows doctors to verify the AI’s reasoning against their medical expertise.
In the financial sector, XAI plays a crucial role in lending decisions. When an AI system denies a loan application, banks must explain the reasoning to their customers. XAI tools make this possible by highlighting which factors, like credit history, income, or debt ratios, most influenced the decision. This transparency helps both bankers and customers understand and trust the process.
Beyond building trust, XAI serves as a vital tool for detecting harmful biases in AI systems. Researchers can use XAI techniques to uncover if a hiring algorithm unfairly favors certain demographic groups or if a medical diagnostic system performs less accurately for specific populations. By making these biases visible, organizations can take steps to create fairer AI systems.
Regulatory compliance represents another key benefit of XAI. As governments worldwide implement stricter rules about AI transparency, organizations must be able to explain their AI systems’ decisions. In the European Union, for instance, citizens have a “right to explanation” when AI systems make decisions affecting them. XAI provides the tools needed to meet these regulatory requirements while maintaining the powerful benefits of AI technology.
Challenges with Black Box Models
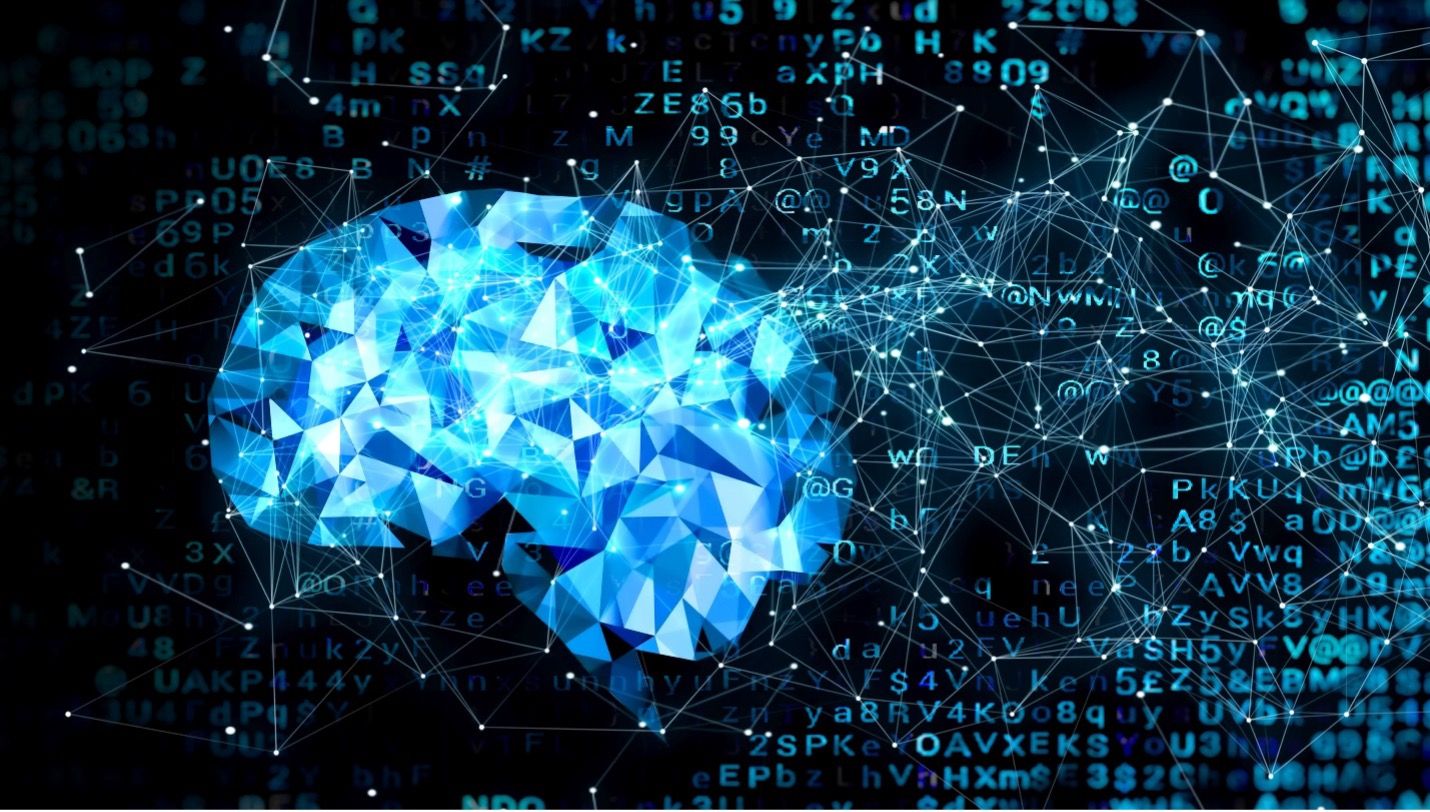
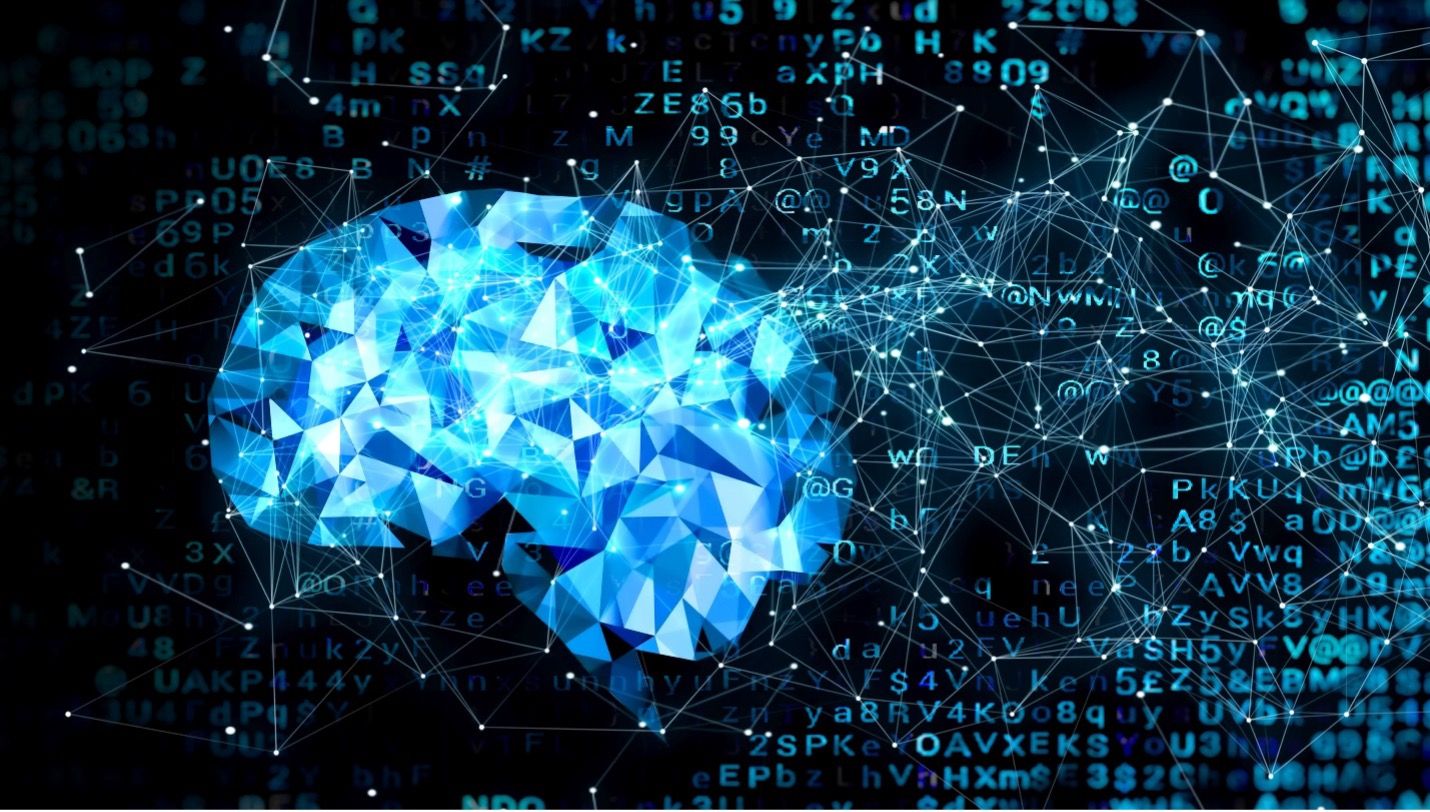
Artificial intelligence systems often use complex algorithms that work like mysterious black boxes—data goes in, decisions come out, but no one can see what happens inside. This lack of transparency creates serious problems, especially when these systems make important decisions about people’s lives.
One major challenge is that black box models can hide harmful biases. For example, in 2018, Amazon discovered their AI hiring tool discriminated against women for technical roles. The system had learned biased patterns from historical data, but because they couldn’t understand how it made decisions, they struggled to fix the problem and eventually had to abandon the tool.
Another critical issue is the difficulty in debugging these systems when things go wrong. When a black box model makes a mistake, data scientists often can’t pinpoint why it happened or how to prevent similar errors in the future. This becomes especially concerning in fields like healthcare, where AI errors could affect patient safety and treatment decisions.
Regulatory compliance poses yet another significant challenge. Many industries now have strict rules requiring companies to explain their automated decisions. For instance, insurance companies must justify why they approve or deny claims. But when using black box models, providing clear explanations becomes nearly impossible, putting companies at risk of violating these regulations.
Trust also suffers when decisions can’t be explained. Imagine being denied a loan or insurance coverage by an AI system, but neither you nor the bank can understand why. This lack of transparency makes it difficult for people to trust these systems or challenge potentially unfair decisions.
Perhaps most troubling is that many organizations use black box models even when simpler, more transparent alternatives could work just as well. Research has shown that in many cases, interpretable models can achieve the same accuracy as complex black box systems while providing clear explanations for their decisions.
Techniques for Implementing Explainable AI
AI systems make countless decisions that affect our daily lives, from healthcare diagnoses to loan approvals. But how can we trust these decisions if we don’t understand how they’re made? Explainable AI techniques shed light on the inner workings of these complex systems.
One widely used method is LIME (Local Interpretable Model-agnostic Explanations). This technique creates simplified explanations for individual predictions by analyzing how changes in input data affect the model’s output. Think of LIME as a translator that converts complex AI decisions into simple, human-friendly explanations.
SHAP (SHapley Additive exPlanations) offers another powerful approach to understanding AI decisions. Unlike LIME, SHAP looks at the entire dataset to determine how each feature contributes to the final prediction. This method assigns importance values to different features, helping us understand which factors most influence the AI’s decision-making process.
Counterfactual explanations provide another perspective by showing how different inputs would change the AI’s output. For example, in a loan approval system, it might explain that increasing your credit score by 50 points would change the decision from rejection to approval. This practical approach helps users understand what they need to change to achieve their desired outcome.
These techniques work together to make AI more transparent and trustworthy. By implementing them, organizations can build AI systems that not only make accurate decisions but also explain their reasoning in clear, understandable terms. This transparency is crucial for building trust between AI systems and the humans who use them.
The aim of XAI at a global level is to explain the behavior of the model across the entire dataset. It gives insights into the main factors influencing the model, and the overall trends and patterns observed.
Whether you’re developing healthcare diagnostics or financial risk assessments, these explainable AI techniques provide the tools needed to create more accountable and transparent AI systems. The future of AI isn’t just about making smart decisions – it’s about making decisions we can understand and trust.
Applications of Explainable AI
Explainable AI (XAI) has emerged as a transformative technology across various industries, enhancing decision-making processes while maintaining transparency. Let’s explore how different sectors leverage XAI to improve their operations and build trust.
In healthcare, XAI helps doctors understand why AI systems make specific diagnostic recommendations. For instance, when an AI system analyzes medical images to detect potential tumors, it can highlight the features in the scan that raised concerns, allowing doctors to verify the AI’s reasoning. According to a study in BMC Medical Informatics and Decision Making, this transparency is crucial for building trust between healthcare professionals and AI systems.
The financial sector uses XAI to revolutionize lending decisions and fraud detection. When a loan application is approved or denied, XAI can break down the factors influencing the decision, such as credit history and income stability. This transparency helps both bankers and customers understand the reasoning behind financial decisions, reducing disputes and improving customer satisfaction.
Legal professionals employ XAI to enhance their decision-making processes. For example, when analyzing legal documents, XAI systems can explain which specific phrases or clauses triggered certain recommendations, aiding lawyers in making more informed decisions about case strategy. This transparency is particularly valuable for complex regulatory compliance issues.
Beyond explaining decisions, XAI helps organizations identify and eliminate potential biases in their AI systems. For example, if an AI system shows unexpected patterns in hiring recommendations, XAI tools can pinpoint which data points are causing these patterns, allowing companies to correct any unfair practices before they impact individuals.
The power of XAI lies not just in its ability to explain decisions but in how it transforms the relationship between humans and AI systems. By making artificial intelligence more transparent and accountable, XAI helps build the trust necessary for wider adoption of AI technologies across all sectors.
Future of Explainable AI
The next chapter of artificial intelligence will be defined by its ability to explain itself. As AI systems become more sophisticated and integrated into our daily lives, the demand for transparency and understanding grows ever stronger. Research teams worldwide are making significant strides in developing AI models that can clearly communicate their decision-making processes while maintaining high performance.
The evolution of explainable AI will bring unprecedented levels of transparency to automated systems. This transparency isn’t just a technical achievement – it’s a fundamental requirement for building trust between humans and AI. When organizations can fully understand how their AI makes decisions, they can ensure those decisions align with ethical principles and regulatory requirements.
Future advancements in XAI will focus on making AI systems more reliable and robust. Research shows that XAI aims to increase the trustworthiness and accountability of AI systems, especially in high-stakes applications like healthcare where lives may depend on the accuracy of AI decisions.
We’ll see growing emphasis on creating AI models that are not only powerful but also inherently interpretable. Rather than treating explainability as an add-on feature, developers will build transparency into AI systems from the ground up. This shift will help ensure that AI remains both capable and comprehensible as it tackles increasingly complex challenges.
The future success of AI depends on striking the right balance between performance and explainability. As we continue to push the boundaries of what AI can achieve, maintaining transparency and ethical considerations will be crucial. This commitment to explainable AI will help create a future where artificial intelligence serves humanity while remaining accountable, trustworthy, and aligned with our values.
Last updated:
Disclaimer: The information presented in this article is for general informational purposes only and is provided as is. While we strive to keep the content up-to-date and accurate, we make no representations or warranties of any kind, express or implied, about the completeness, accuracy, reliability, suitability, or availability of the information contained in this article.
Any reliance you place on such information is strictly at your own risk. We reserve the right to make additions, deletions, or modifications to the contents of this article at any time without prior notice.
In no event will we be liable for any loss or damage including without limitation, indirect or consequential loss or damage, or any loss or damage whatsoever arising from loss of data, profits, or any other loss not specified herein arising out of, or in connection with, the use of this article.
Despite our best efforts, this article may contain oversights, errors, or omissions. If you notice any inaccuracies or have concerns about the content, please report them through our content feedback form. Your input helps us maintain the quality and reliability of our information.