Building Trust in Human-AI Collaboration: Key Strategies for Success
Imagine a world where artificial intelligence is not just a tool, but a trusted teammate. As developers and technical leaders push the boundaries of AI capabilities, one critical question emerges: How do we build genuine trust between humans and AI systems?
The intersection of human intelligence and AI capabilities presents both extraordinary opportunities and unique challenges. Recent research has revealed that successful human-AI partnerships hinge on multiple factors, including perceived rapport, environmental conditions, and perhaps most crucially, the development of appropriate trust levels.
Trust is not just a feel-good metric – it is the foundation that determines whether AI systems will be embraced or abandoned. When trust is calibrated correctly, teams flourish. But when trust falters, we risk either over-reliance on AI systems or their complete disuse, neither of which serves our goals for advancement.
Throughout this exploration, we will uncover the nuanced dynamics of human-AI collaboration, examining how trust evolves over time and what factors influence its development. From transparency in AI decision-making to the role of user confidence, we will dive deep into the elements that make or break these crucial partnerships.
Whether you are building autonomous systems or integrating AI into existing workflows, understanding the trust equation is not just helpful – it is essential for creating AI solutions that truly serve their purpose while maintaining user confidence and engagement. Let us explore how to bridge the gap between human intuition and artificial intelligence, creating partnerships that stand the test of time.
Building Effective Human-AI Teams
The dynamics of human-AI collaboration are evolving, challenging traditional teamwork and productivity concepts. Recent MIT Sloan research highlights that successful human-AI partnerships leverage each party’s unique strengths rather than blindly integrating them.
AI excels in decision-making tasks like medical diagnoses or data analysis by processing vast amounts of information accurately. However, humans bring irreplaceable qualities such as context understanding, emotional intelligence, and creative thinking in unprecedented situations. The goal is to find the sweet spot where these complementary abilities enhance each other.
Interestingly, the research suggests that human-AI teams excel in creative endeavors. While AI handles routine aspects and generates initial ideas, humans refine these suggestions, adding emotional depth and ensuring the final output resonates with the audience. This synergy is valuable in content creation, design work, and problem-solving scenarios requiring both analytical precision and creative insight.
Building effective human-AI teams is not just about technology but also fostering the right mindset. Thriving teams see AI as a collaborative tool to augment human capabilities, not replace human judgment.
Successful implementation requires clear protocols and expectations. Organizations must establish frameworks defining when to rely on AI analysis and when to prioritize human insight. This might involve using AI for initial data processing and pattern recognition while reserving final decisions and creative direction for human team members. Through this balanced approach, human-AI teams can achieve outcomes neither could accomplish alone.
Challenges in Human-AI Trust
Building trust between humans and artificial intelligence systems presents unique hurdles that extend beyond typical technology adoption barriers. Recent research has shown that AI’s unpredictable behavior creates significant trust challenges, particularly because humans naturally seek predictability when forming trust relationships.
Unlike trusting a human colleague or friend, where we can understand their reasoning and motivations, AI systems often operate as black boxes. Their decision-making processes, involving trillions of parameters, remain largely opaque even to their creators. This lack of transparency makes it difficult for users to develop the confidence needed for meaningful collaboration.
Past experiences with technology significantly shape how users approach AI systems. Those who have encountered misleading or unreliable AI outputs tend to maintain a heightened sense of skepticism. For instance, when an AI makes an unexpected or incorrect decision, users often find it harder to rebuild trust compared to human errors, since machines cannot explain their reasoning or show genuine accountability.
The challenge becomes more complex in critical applications like healthcare or financial services, where the stakes are particularly high. Medical professionals, for example, must carefully balance the potential benefits of AI diagnostic tools against their inherent unpredictability. This creates a constant tension between leveraging AI’s capabilities and maintaining appropriate caution.
The varying levels of technical literacy among users further complicate trust-building efforts. While tech-savvy individuals might better understand AI’s limitations and capabilities, others may either over-trust or completely reject AI assistance based on misconceptions. This disparity in understanding creates an additional layer of complexity in designing AI systems that can earn and maintain user trust across different user groups.
Trust is grounded in predictability. It depends on your ability to anticipate the behavior of others. If you trust someone and they don’t do what you expect, then your perception of their trustworthiness diminishes.
Scientific American, 2023
Strategies for Enhancing Trust
Building trust in AI systems demands a methodical approach focused on transparency, fairness, and consistent performance. Recent studies from KPMG reveal that 61% of people remain wary about trusting AI decisions, highlighting the critical need for organizations to implement robust trust-building strategies.
Transparency stands as the cornerstone of trustworthy AI. Organizations must provide clear explanations of how their AI systems collect, process, and utilize data to make decisions. As leading industry experts emphasize, this involves documenting data sources, model architectures, and validation processes throughout the entire AI lifecycle.
Fairness in AI operations requires rigorous testing and mitigation of potential biases. Organizations should implement comprehensive bias detection protocols and ensure diverse teams are involved in AI development. This approach helps prevent discriminatory outcomes while maintaining the system’s ethical integrity. Regular audits and assessments play a crucial role in identifying and addressing any fairness concerns that may emerge over time.
System robustness represents another vital element in fostering user confidence. AI systems must demonstrate consistent performance under varying conditions, with clear protocols for handling errors or unexpected situations. This includes implementing fail-safes and maintaining detailed logs of system actions for accountability purposes.
Clear communication channels between AI developers and users prove essential for maintaining trust. Organizations should provide accessible documentation and regular updates about system capabilities and limitations. This transparency helps users understand what to expect from the AI system and how to interpret its outputs effectively.
Regular performance monitoring and validation ensure that AI systems continue to meet user expectations and maintain their trustworthiness over time. This involves tracking key metrics, gathering user feedback, and making necessary adjustments to improve system reliability and accuracy.
Metric | Description |
---|---|
Accuracy | Measures the proportion of correct predictions out of the total predictions made by the model. |
Precision | Measures the proportion of true positive predictions among all positive predictions made by the model. |
Recall | Measures the proportion of true positive predictions among all actual positive instances in the dataset. |
F1 Score | Combines precision and recall to provide a balanced measure of a model’s performance. |
Confusion Matrix | Summarizes the performance of a classification model by showing the counts of true positives, true negatives, false positives, and false negatives. |
ROC Curve and AUC | ROC curve plots the true positive rate against the false positive rate, and AUC measures the area under the ROC curve. |
Cross-Validation | Technique to assess model performance by training and testing on different subsets of data. |
Building trust in AI will require a significant effort to instill in it a sense of morality, operate in full transparency and provide education about the opportunities it will create for business and consumers.
Success in building trust requires ongoing commitment to these strategies, coupled with regular assessment and refinement of trust-building measures. Organizations that prioritize transparency, fairness, and robust performance position themselves to earn and maintain user confidence in their AI systems.
The Role of Monitoring in Human-AI Systems
Continuous monitoring is crucial for trustworthy human-AI collaboration. As AI systems integrate into critical operations, vigilant oversight ensures reliable performance and adaptation to real-world challenges. Regular monitoring identifies potential issues before they impact performance, fostering trust between human operators and AI technology.
Systematic monitoring covers several key aspects of AI performance. According to research on AI monitoring best practices, organizations must track key performance indicators, implement anomaly detection, and maintain robust data integrity checks. These measures ensure AI systems operate within expected parameters and deliver consistent results.
User feedback is instrumental in refining AI performance. Real-time user input helps developers quickly identify areas needing improvement and implement necessary adjustments. This iterative feedback loop aligns AI capabilities with user expectations and operational requirements, enhancing human-AI collaboration.
System updates are another critical aspect of effective AI monitoring. Regular updates address technical issues and incorporate learnings from user interactions and emerging challenges. These updates help prevent concept drift—where AI models become less accurate over time as real-world conditions change. Maintaining current and optimized systems ensures AI tools remain reliable and effective.
Monitoring also includes ethical considerations and user trust. Organizations must establish protocols for reviewing AI decisions and implementing safeguards against biases or errors. This comprehensive approach maintains transparency and accountability in human-AI interactions, essential for building lasting trust in these systems.
Regular audits, both internal and third-party, help ensure that AI systems meet established performance, compliance, and ethical standards.
Robust monitoring practices help organizations maintain system integrity and foster user confidence. By combining continuous technical oversight with responsive user feedback, organizations can create resilient and trustworthy human-AI partnerships that deliver consistent value over time.
Practice | Description |
---|---|
Continuous Monitoring | Implement real-time monitoring to track performance metrics and alert teams to deviations or anomalies. |
Performance Metrics | Define and regularly review KPIs such as accuracy, precision, recall, and F1 score. |
Anomaly Detection | Set up systems to identify unusual patterns that indicate performance issues or security threats. |
Data Integrity | Ensure data quality and consistency through regular checks and corrections. |
Model Drift | Monitor for model drift and retrain models periodically to maintain accuracy. |
Explainability and Transparency | Use tools to enhance transparency in AI decision-making and document decision logic. |
Feedback Loops | Establish feedback loops for continuous improvement based on user or system feedback. |
Security and Privacy | Implement robust security measures and comply with data privacy regulations. |
Regular Audits | Conduct internal and third-party audits to ensure compliance with performance, compliance, and ethical standards. |
Incident Management | Develop and train teams on incident management plans to address AI system failures. |
Documentation and Reporting | Maintain detailed documentation and reporting of AI system performance and monitoring activities. |
How SmythOS Supports Trust in Human-AI Collaboration
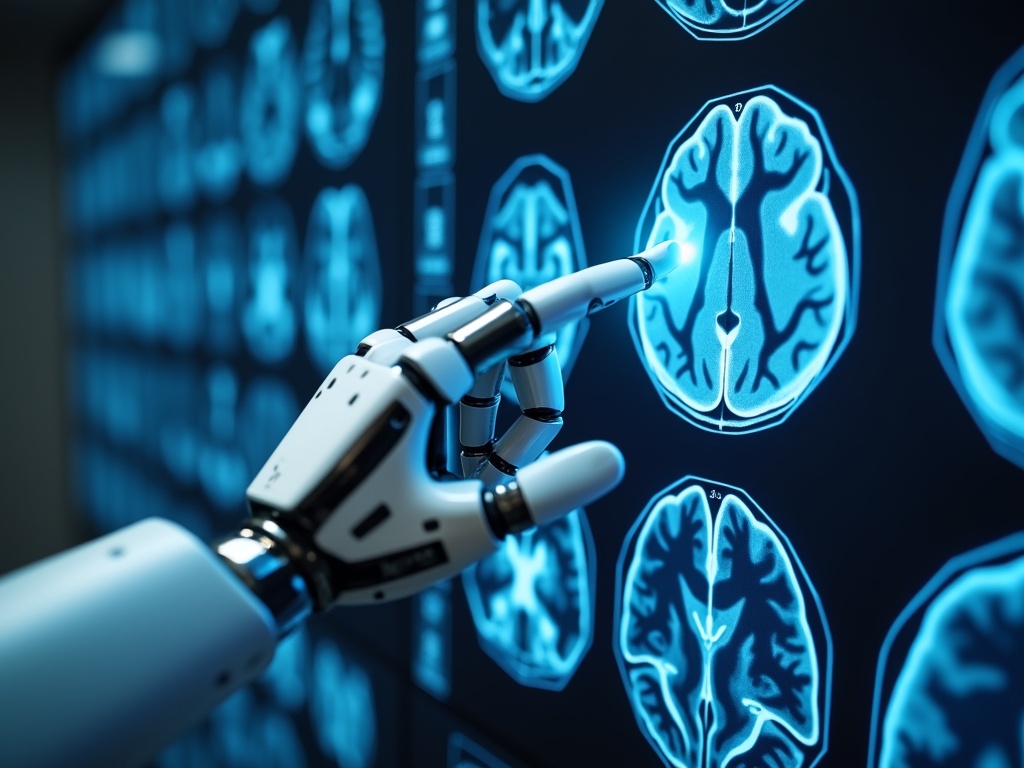
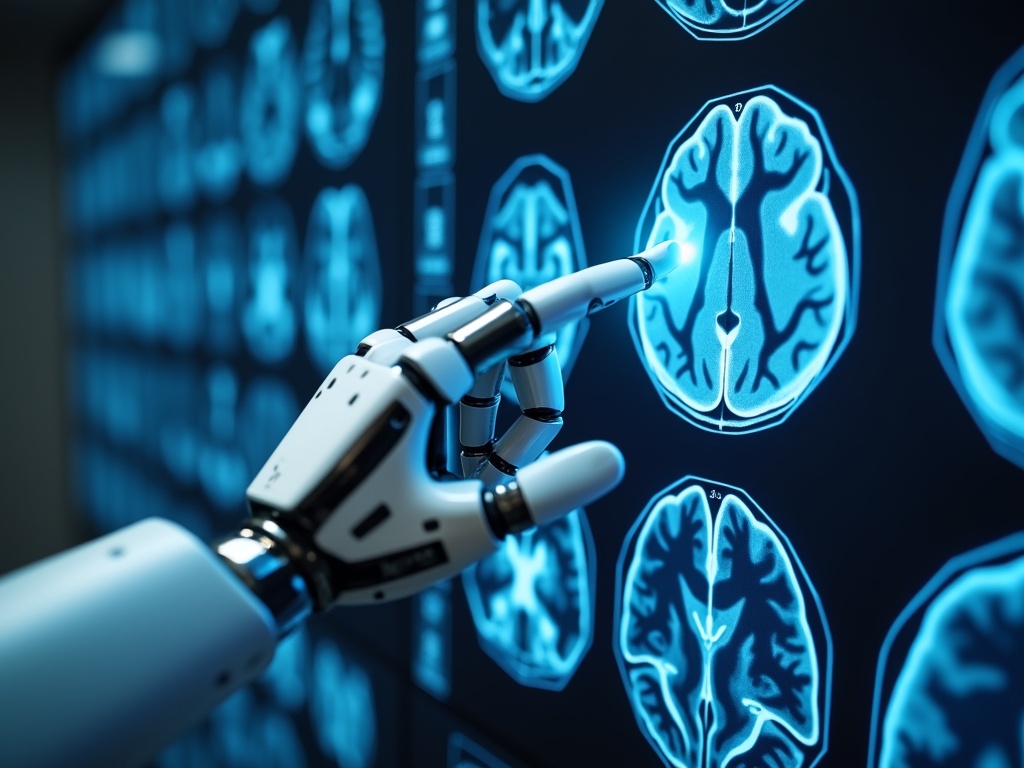
Robotic hand pointing at illuminated brain scans.
Building trust between humans and artificial intelligence systems remains a critical challenge. SmythOS addresses this through its comprehensive approach to secure, transparent AI operations. The platform’s robust monitoring capabilities continuously track AI agent behavior and performance to ensure systems operate within defined parameters.
At the core of SmythOS’s trust-building framework lies its sophisticated integration system. Unlike conventional platforms that treat AI as a separate tool, SmythOS seamlessly connects AI agents with existing business tools and workflows. As noted by industry experts, this unified approach enables businesses to maintain oversight while leveraging AI’s full potential.
The platform’s built-in monitoring tools provide unprecedented visibility into AI operations. Through an intuitive dashboard, teams can track critical performance metrics in real-time, swiftly identify potential issues, and optimize resource allocation before problems arise. This proactive monitoring ensures AI systems maintain peak efficiency while operating within established ethical and security boundaries.
Security stands as another paramount feature in SmythOS’s trust-enhancement arsenal. The platform implements military-grade encryption protocols and rigorous data validation processes to protect sensitive information. This comprehensive security framework ensures that all AI-human interactions remain confidential and protected from potential threats.
SmythOS isn’t just about building AI – it’s about building trust. Our platform ensures that your AI agents are not only intelligent but also impenetrable to those who would seek to compromise them.
Beyond technical safeguards, SmythOS emphasizes transparency in AI decision-making. The platform’s visual debugging environment allows teams to inspect and understand AI workflows, demystifying the often opaque nature of artificial intelligence. This transparency helps build confidence among users, as they can clearly see how AI agents make decisions and process information.
Through these comprehensive trust-building features, SmythOS creates an environment where humans and AI can collaborate effectively while maintaining security, reliability, and transparency. This thoughtful approach to human-AI partnership paves the way for more productive and trustworthy artificial intelligence implementations across organizations.
Future Directions in Human-AI Trust
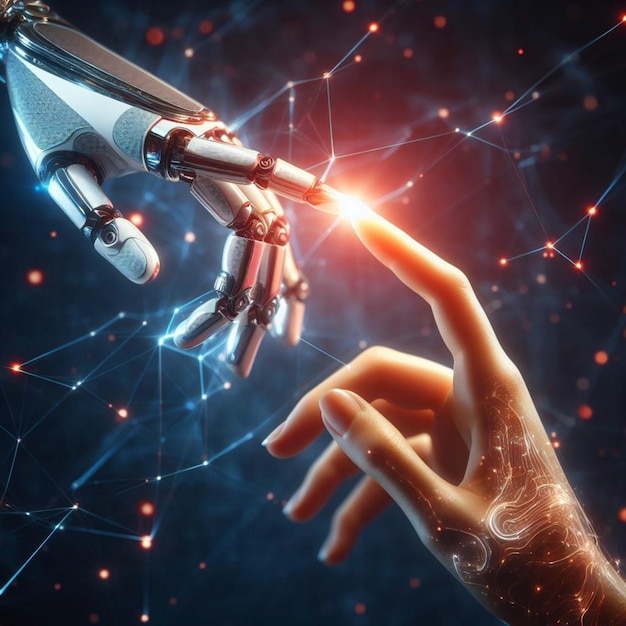
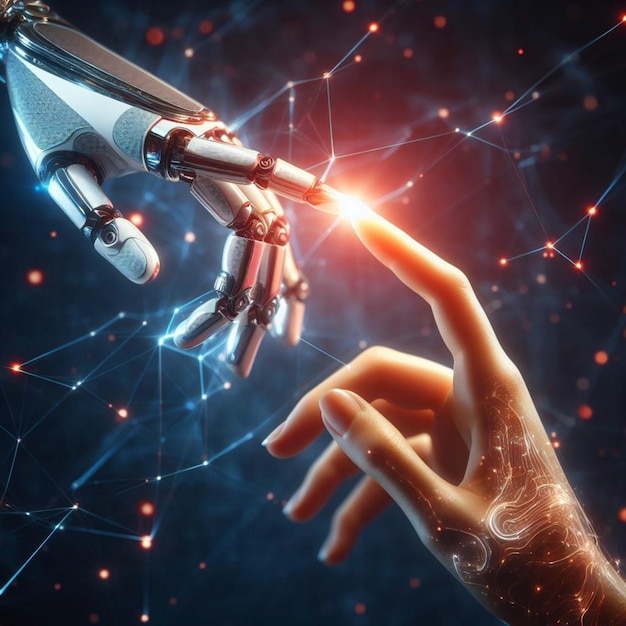
A symbol of collaboration between humans and AI. – Via freepik.com
The future landscape of human-AI collaboration hinges on establishing deeper foundations of trust and developing more sophisticated cooperation frameworks. As organizations increasingly deploy AI systems, the focus must shift from purely technical capabilities to nurturing genuine partnerships between human operators and AI agents. Current challenges around transparency and reliability need systematic solutions that bridge the gap between AI’s analytical power and human intuition.
A significant trend emerging in this space is the development of bi-directional trust mechanisms. Rather than viewing trust as a one-way street where humans simply verify AI outputs, next-generation systems will incorporate what researchers call “active trust management” – a dynamic process where both human and AI team members continuously assess and calibrate their trust in each other’s capabilities and limitations. This represents a fundamental shift from today’s relatively static trust models.
The integration of physiological measures presents another promising frontier. As highlighted in recent research on human-AI healthcare partnerships, systems that can monitor subtle indicators of human cognitive states – from stress levels to attention patterns – will enable more responsive and context-aware collaboration. This deeper understanding of human partners will allow AI systems to adjust their behavior and communication styles in real-time.
Transparency will evolve beyond simple explanations of AI decisions to include richer forms of knowledge sharing. Future systems will need to communicate not just what they know, but also what they don’t know, expressing uncertainty in ways that human teammates can intuitively grasp and factor into their decision-making. This enhanced transparency will be crucial for building the kind of resilient trust needed in high-stakes environments.
Perhaps most importantly, we’ll see a shift toward what experts call “calibrated trust” – where both humans and AI systems have an appropriate level of skepticism about each other’s capabilities. This balanced approach, neither overly trusting nor overly suspicious, will be essential for creating truly effective human-AI teams that can leverage the unique strengths of both human and artificial intelligence while accounting for their respective limitations.
Last updated:
Disclaimer: The information presented in this article is for general informational purposes only and is provided as is. While we strive to keep the content up-to-date and accurate, we make no representations or warranties of any kind, express or implied, about the completeness, accuracy, reliability, suitability, or availability of the information contained in this article.
Any reliance you place on such information is strictly at your own risk. We reserve the right to make additions, deletions, or modifications to the contents of this article at any time without prior notice.
In no event will we be liable for any loss or damage including without limitation, indirect or consequential loss or damage, or any loss or damage whatsoever arising from loss of data, profits, or any other loss not specified herein arising out of, or in connection with, the use of this article.
Despite our best efforts, this article may contain oversights, errors, or omissions. If you notice any inaccuracies or have concerns about the content, please report them through our content feedback form. Your input helps us maintain the quality and reliability of our information.