Intelligent Agents and Machine Learning: Transforming Automation and Decision-Making
Artificial intelligence is rapidly evolving, with intelligent agents leading the way. These entities are changing how machines interact with and respond to their environments. But what exactly are intelligent agents, and how do they relate to machine learning?
Intelligent agents are AI systems designed to perceive their surroundings, process information, and take autonomous actions to achieve specific goals. They are digital decision-makers, constantly analyzing data and adapting their behaviors to maximize success. Unlike simple programs, these agents can learn from experience, improving their performance over time.
The synergy between intelligent agents and machine learning is transformative. Machine learning algorithms allow agents to recognize patterns, make predictions, and optimize their decision-making processes. This combination is driving advancements across industries, from healthcare diagnostics to financial trading.
Intelligent agents are not just theoretical constructs—they’re already making a real-world impact. Consider a customer service chatbot that truly understands context or a self-driving car that can navigate complex traffic scenarios. These are just glimpses of what intelligent agents can achieve.
Exploring the components, functionalities, and applications of intelligent agents reveals how they revolutionize problem-solving and decision-making in AI. Machines don’t just compute; they think, learn, and adapt in ways that are transforming our technological landscape.
Understanding Intelligent Agents
Intelligent agents represent a fascinating frontier in artificial intelligence, pushing the boundaries of what machines can achieve. These sophisticated AI systems make autonomous decisions and adapt to changing circumstances. Unlike simpler programs that follow rigid instructions, intelligent agents perceive their environment, reason about it, and take actions to achieve specific goals without constant human oversight.
At the core of an intelligent agent’s capabilities are several key components:
- Perception mechanisms that allow the agent to sense and interpret its surroundings
- Reasoning capabilities that enable it to process information and make decisions
- Memory storage to retain knowledge and learn from past experiences
- Learning modules that help the agent improve its performance over time
This intricate interplay of components allows intelligent agents to navigate complex, real-world scenarios with impressive flexibility. Virtual assistants like Siri or Alexa are prime examples. When you ask Siri about the weather, it actively interprets your speech, understands the context, accesses relevant data, and formulates a natural language response in seconds.
What sets intelligent agents apart is their capacity for learning and adaptation. Each interaction refines the agent’s understanding and improves its responses. Over time, a virtual assistant can become more attuned to your specific speech patterns, preferences, and habits, offering an increasingly personalized experience.
As we continue to interact with intelligent agents in various aspects of our lives—from smart home devices to advanced diagnostic tools in healthcare—it’s clear that this technology is reshaping how we engage with digital systems. The autonomous decision-making capabilities of these agents open new possibilities for automation and assistance, promising to make our interactions with technology more intuitive and efficient.
Components of Intelligent Agents
Intelligent agents, the driving force behind cutting-edge AI systems, are composed of several critical components that work together to enable their remarkable functionalities. These components form the backbone of AI’s ability to perceive, reason, remember, and learn – mirroring human cognitive processes in many ways. Let’s explore each of these components and their real-world applications.
Perception Mechanisms: The Agent’s Senses
Perception mechanisms serve as the eyes and ears of an intelligent agent, allowing it to gather data from its environment. In self-driving cars, this component is crucial. These vehicles rely on sensors, including cameras, LIDAR (Light Detection and Ranging), and radar, to ‘see’ the world around them.
Tesla’s Autopilot system, for example, uses eight cameras to provide 360-degree visibility around the car. These cameras can detect everything from other vehicles and pedestrians to traffic signs and road markings. The perception data gathered by these sensors forms the foundation for the agent’s decision-making process.
Sensor Type | Advantages | Disadvantages |
---|---|---|
Camera | Vision-like sensory, Recognizing 2D information, Low cost | Poor vision under extreme weather events |
Radar | Unaffected by weather conditions, Default sensor for emergency braking | Low-definition modeling |
LiDAR | High-definition 3D modeling, Unaffected by weather conditions | Highly sophisticated, High cost |
Reasoning Capabilities: The Brain of the Operation
Once an intelligent agent has gathered data through its perception mechanisms, it needs to make sense of this information. This is where reasoning capabilities come into play. In self-driving cars, sophisticated AI algorithms process the sensor data to interpret the environment and make decisions.
These algorithms use techniques like deep learning and computer vision to identify objects, predict their behavior, and plan the vehicle’s actions. For example, when a self-driving car approaches a crosswalk, its reasoning capabilities allow it to recognize pedestrians, anticipate their movements, and decide whether to stop or proceed.
Memory Storage: Learning from Experience
Just as humans learn from past experiences, intelligent agents need a way to store and recall information. Memory storage components allow agents to retain data about past events, decisions, and outcomes. In autonomous vehicles, this often takes the form of extensive data logs.
Tesla, for instance, has been collecting billions of miles worth of driving data from its vehicles. This massive dataset serves as a collective memory, informing future decision-making and helping to improve the overall system. When a Tesla encounters a new situation, it can draw upon this vast repository of past experiences to inform its actions.
Learning Modules: Continuous Improvement
Perhaps the most fascinating aspect of intelligent agents is their ability to learn and improve over time. Learning modules enable agents to refine their decision-making processes based on new experiences and feedback. In the world of self-driving cars, this manifests as over-the-air updates that enhance the vehicle’s capabilities.
Waymo, Alphabet’s self-driving car project, uses machine learning algorithms that allow its vehicles to continually improve their driving skills. These algorithms analyze the data collected from millions of miles of real-world and simulated driving, identifying patterns and refining the vehicle’s responses to various scenarios.
By integrating these four components – perception, reasoning, memory, and learning – intelligent agents can navigate complex environments, make informed decisions, and continuously evolve. As we continue to refine these technologies, we move closer to a future where AI can match and even surpass human capabilities in specific domains.
Applications of Intelligent Agents
Intelligent agents are enhancing operational efficiency and decision-making across various industries. These AI-powered entities are finding applications in sectors such as healthcare, finance, and customer service, proving their versatility and value.
In healthcare, intelligent agents are making significant strides in diagnostic assistance. By analyzing vast amounts of medical data, including patient histories, symptoms, and test results, these agents can help doctors make more accurate diagnoses in less time. For instance, an AI agent might flag potential issues in a chest X-ray that a human radiologist could overlook, leading to earlier detection and treatment of diseases.
The finance industry has embraced intelligent agents, particularly in trading. AI-powered trading algorithms can process market data at lightning speed, identifying trends and executing trades faster than any human trader. These agents don’t just react to market changes; they can predict them, giving firms a competitive edge. As one financial analyst put it:
AI trading agents have transformed the market. They’re not just faster; they’re smarter, able to spot patterns we humans might miss. It’s like having a tireless, genius trader working 24/7.
Jane Doe, Financial Analyst at Top Tier Investments
Customer service is another area where intelligent agents are making waves. Chatbots powered by natural language processing can handle customer inquiries around the clock, providing instant responses and freeing up human agents for more complex issues. These AI assistants can learn from each interaction, continually improving their ability to understand and respond to customer needs.
The benefits of implementing intelligent agents are clear: reduced costs, increased productivity, and improved outcomes. By automating complex tasks, these agents allow businesses to operate more efficiently and effectively. For example, a customer service chatbot can handle hundreds of inquiries simultaneously, drastically reducing wait times and improving customer satisfaction.
As intelligent agents continue to evolve, we can expect to see even more innovative applications across industries. From personalized healthcare recommendations to predictive maintenance in manufacturing, the potential of these AI-powered assistants is vast. The key for businesses will be identifying where intelligent agents can add the most value and integrating them thoughtfully into existing processes.
The Future of Work with Intelligent Agents
Looking to the future, intelligent agents will play an increasingly important role in the workplace. Rather than replacing human workers, these AI assistants are more likely to augment human capabilities, taking on repetitive or data-intensive tasks and freeing up people to focus on more creative and strategic work.
For example, in a law firm, intelligent agents might be used to review contracts, flagging potential issues for human lawyers to address. This not only speeds up the review process but also reduces the risk of human error. Similarly, in marketing, AI agents could analyze consumer data to identify trends and suggest campaign strategies, with human marketers providing the creative direction.
The key to successfully integrating intelligent agents into the workforce will be striking the right balance between AI capabilities and human expertise. As one tech expert notes:
The most successful companies won’t be those that simply deploy the most AI, but those that figure out how to best combine human and artificial intelligence.
As we continue to explore the potential of intelligent agents, it’s crucial to consider not just their technical capabilities, but also the ethical implications of their use. Questions about data privacy, decision-making transparency, and the potential for bias in AI systems will need to be addressed as these technologies become more prevalent.
Intelligent agents are not just a futuristic concept; they’re here now, transforming industries and reshaping the way we work. From improving healthcare outcomes to revolutionizing financial trading to enhancing customer service, these AI-powered assistants are proving their worth across a wide range of applications. As the technology continues to advance, the potential for intelligent agents to drive innovation and efficiency seems boundless.
Challenges of Intelligent Agents
Intelligent agents offer tremendous potential to improve decision-making across industries, but they also face significant hurdles. These AI-powered systems grapple with complex challenges around data privacy, bias management, and algorithmic transparency—issues central to ethical AI development.
One major concern is protecting sensitive data used to train and operate intelligent agents. As these systems ingest massive datasets to learn and make predictions, they risk exposing personal information or proprietary business data. Companies deploying AI must implement robust data governance frameworks and security measures to safeguard privacy. This challenge is especially acute in fields like healthcare, where intelligent agents may handle confidential patient records.
Bias in AI decision-making is another critical issue. Intelligent agents can inadvertently perpetuate and amplify societal biases present in their training data or embedded in their algorithms. For instance, AI-powered hiring tools have come under scrutiny for potentially discriminating against women and minority candidates. As NIST researcher Reva Schwartz notes, “Many of these factors go beyond the technology itself to the impacts of the technology.” Addressing bias requires examining the broader societal context in which these systems operate.
Example | Description | Impact |
---|---|---|
Healthcare | Underrepresented data of women or minority groups can skew predictive AI algorithms. | Lower accuracy results for black patients than white patients in CAD systems. |
Applicant Tracking Systems | Issues with natural language processing algorithms can produce biased results. | Amazon’s hiring algorithm favored male applicants based on specific keywords. |
Online Advertising | Biases in search engine ad algorithms can reinforce job role gender bias. | Google’s system displayed high-paying positions to males more often than to females. |
Image Generation | Generative AI art applications can show gendered bias. | Midjourney depicted older individuals as men in specialized professions. |
Predictive Policing Tools | Relying on historical arrest data can reinforce racial profiling. | Disproportionate targeting of minority communities. |
Algorithmic transparency is another challenge. As intelligent agents become more sophisticated, their decision-making processes grow increasingly opaque. This “black box” nature makes it difficult to audit systems for fairness or identify the root causes of biased outcomes. There is a growing push for “explainable AI” that can articulate the reasoning behind its decisions in human-understandable terms.
Managing these ethical considerations requires ongoing monitoring and refinement of intelligent agents. Organizations must establish governance structures and review processes to continually assess AI systems for unintended consequences or divergence from intended behaviors. This may involve assembling diverse, multidisciplinary teams to evaluate intelligent agents from different perspectives.
Ultimately, realizing the potential of intelligent agents while mitigating risks requires a holistic, socio-technical approach. As NIST advocates, this means expanding our view beyond purely technical solutions to consider AI’s broader societal impact. By proactively addressing challenges around privacy, bias, and transparency, we can work towards building AI systems worthy of the public’s trust.
The Future of Intelligent Agents and Machine Learning
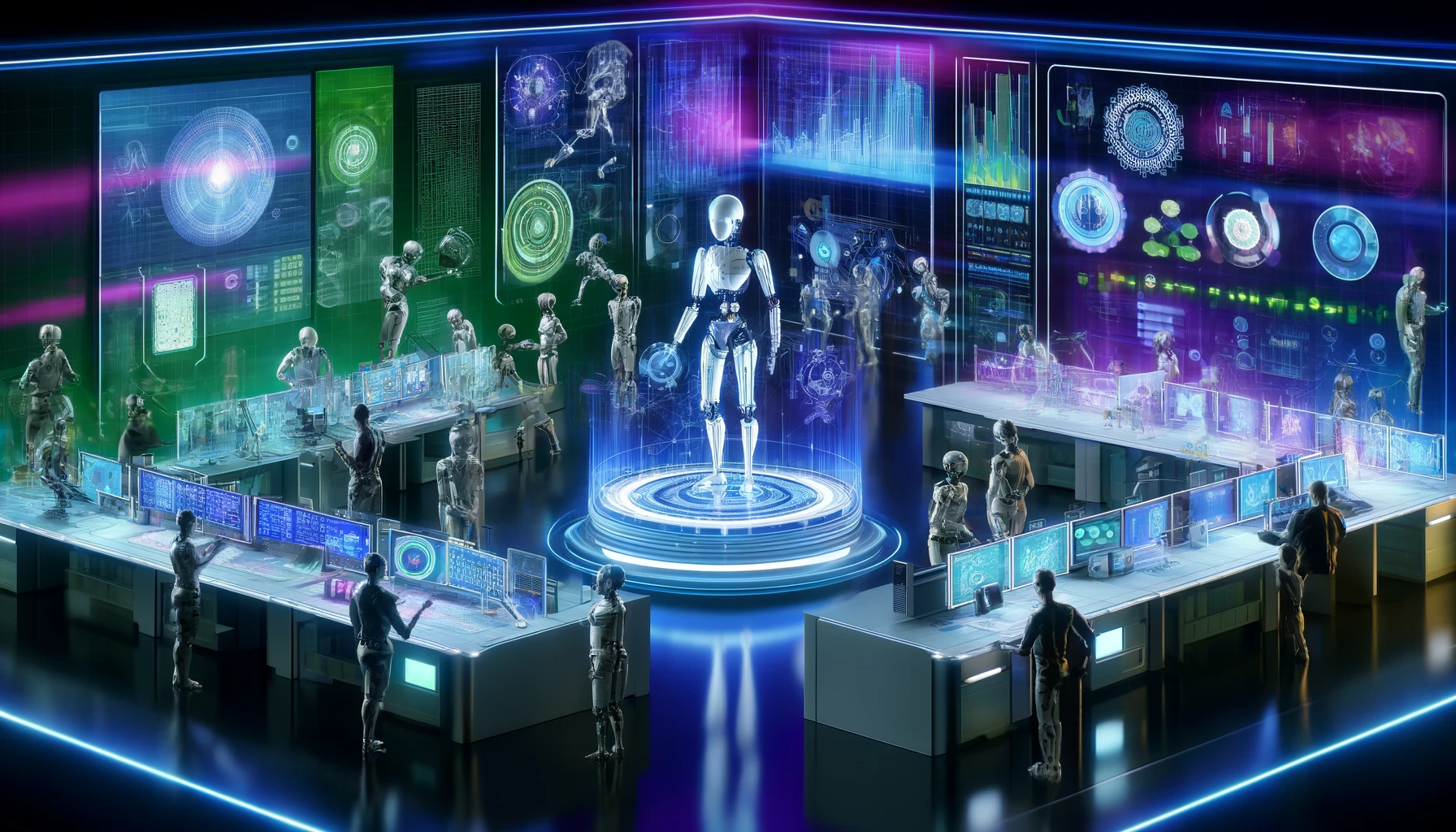
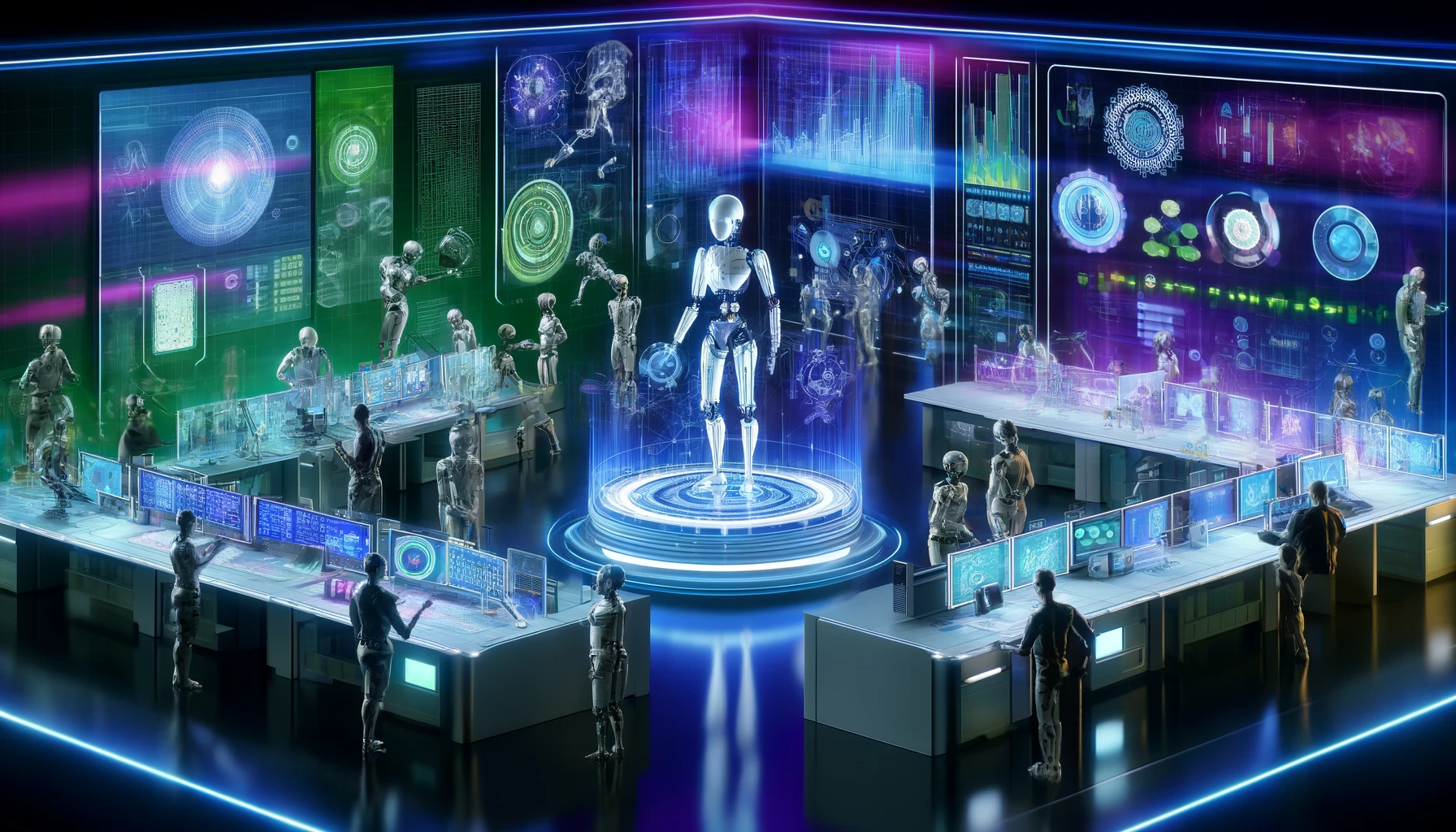
The future of intelligent agents promises to revolutionize how we interact with technology and solve complex problems. These digital entities, powered by sophisticated machine learning algorithms, are poised to tackle increasingly intricate tasks with unprecedented efficiency and accuracy.
Advancements in machine learning are propelling intelligent agents to new heights of capability. We’re witnessing the emergence of AI systems that can not only process vast amounts of data but also learn and adapt in real-time, mimicking human-like decision-making processes. This leap forward is set to enhance automation across industries, from manufacturing to healthcare, streamlining operations and reducing human error.
Perhaps most exciting is the potential for hyper-personalization. As intelligent agents become more adept at understanding context and individual preferences, we can expect tailored experiences in every aspect of our lives. Imagine a world where your digital assistant anticipates your needs before you even express them, or where autonomous vehicles negotiate traffic patterns with the finesse of seasoned drivers.
At the forefront of this AI transformation stands SmythOS, a platform that democratizes the development and deployment of intelligent agents. By offering intuitive tools and a visual workflow builder, SmythOS empowers organizations of all sizes to harness the power of AI without extensive coding expertise. This accessibility is crucial in driving innovation and operational excellence across diverse sectors.
As we look to the future, the symbiosis between human creativity and machine intelligence will likely redefine the boundaries of what’s possible. Platforms like SmythOS are not just facilitating this transformation; they’re accelerating it, paving the way for a new generation of AI-driven solutions that promise to tackle some of our most pressing global challenges.
The question isn’t whether intelligent agents will transform our world, but how quickly we’ll adapt to harness their full potential. As we embrace this AI-driven future, one thing is clear: the organizations that leverage platforms like SmythOS to develop and deploy intelligent agents will be at the vanguard of innovation, shaping industries and driving progress in ways we’re only beginning to imagine.
Last updated:
Disclaimer: The information presented in this article is for general informational purposes only and is provided as is. While we strive to keep the content up-to-date and accurate, we make no representations or warranties of any kind, express or implied, about the completeness, accuracy, reliability, suitability, or availability of the information contained in this article.
Any reliance you place on such information is strictly at your own risk. We reserve the right to make additions, deletions, or modifications to the contents of this article at any time without prior notice.
In no event will we be liable for any loss or damage including without limitation, indirect or consequential loss or damage, or any loss or damage whatsoever arising from loss of data, profits, or any other loss not specified herein arising out of, or in connection with, the use of this article.
Despite our best efforts, this article may contain oversights, errors, or omissions. If you notice any inaccuracies or have concerns about the content, please report them through our content feedback form. Your input helps us maintain the quality and reliability of our information.